Terms like “Machine Learning” and “Big data” are among the most persistent buzzwords of any tech newsfeed right now and for a reason. This is due to the success of A.I. technologies and automatic decision-making that allow the development of robust and autonomous intelligent applications. Both, revolutionize business operations by increasing revenue, lowering costs, improving the processes, and providing lots of new opportunities.
In recent years, all sectors of the economy have been engaging in the usage of Machine Learning and Big Data in order to optimize their results. Similarly, in debt collections the same process applies, Banks and credit institutions, are always looking for a way to increase collectability and lower collections costs. But how can ML and Big Data help in this direction?
WHAT IS MACHINE LEARNING AND BIG DATA?
In -very- simple terms, Machine Learning is the science of getting computers to act without being explicitly programmed by a human to do so. The actions of the computer are based on rules that it learned -through algorithms- from some data. These data are usually large and complex datasets, thus the term "Big".
Machine Learning is a pretty old -in terms of current technology pace- field, dating back to the 1950s. Big data, on the other hand, became a thing around 2013 when it was discovered that 90% of the data in the world at that point, was produced during the previous 2 years. During the last years, these large volumes of data, new engineering insights, and powerful hardware gave the ability to Machine Learning to produce useful results in reasonable time and revealed this way its true potential.
The nowadays multi-dimensional nature of a customers’ characteristics, the subjective judgment of financial analysts (i.e., ad hoc decisions based on experience), as well as the complexity that occurs from designing with high predictive performance, necessitate the interaction of financial risk management with scientific areas such as statistics, machine learning, operations research and decision making. How to capture reliable, valuable, and accurate information in massive data is one of the most significant research topics nowadays.
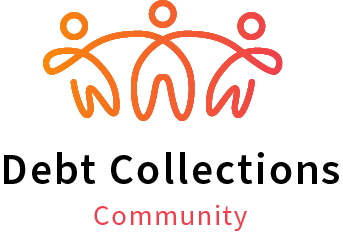
The debt collections community was built for the sole purpose of connecting professionals from the debt collections industry. CROs, credit risk officers, debt collections & recovery managers, supervisors and team leaders with a minimum of 3 years of experience in managing credit risk and delinquent portfolios, within financial institutions (banks, consumer finance, microfinance, leasing, debt services/buyers, etc.), utilities, telcos , or debt collections agencies are eligible to join.
Collections challenges in the A.I. era
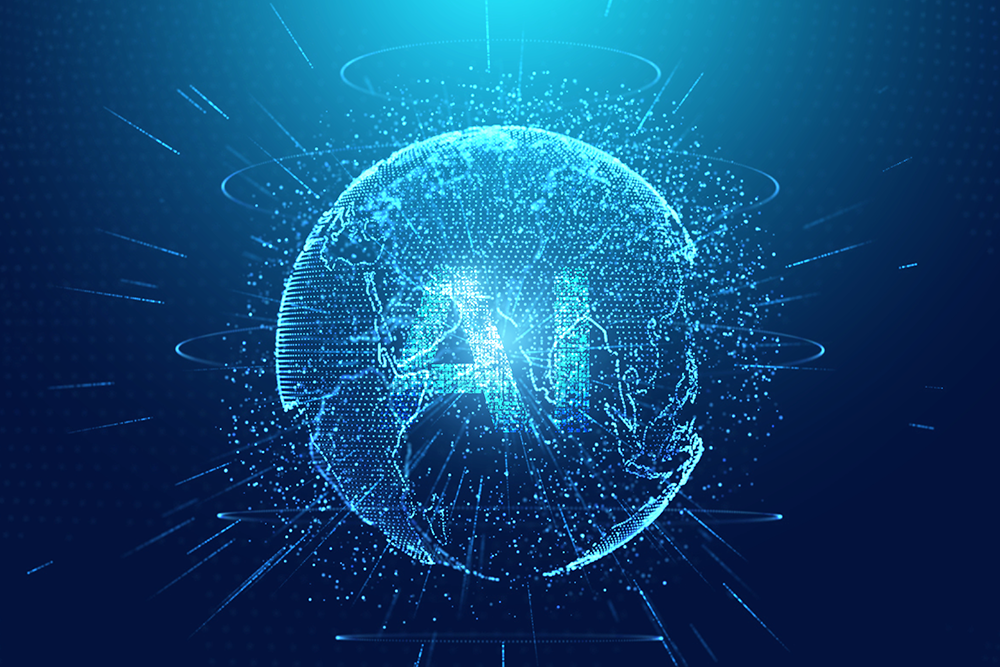
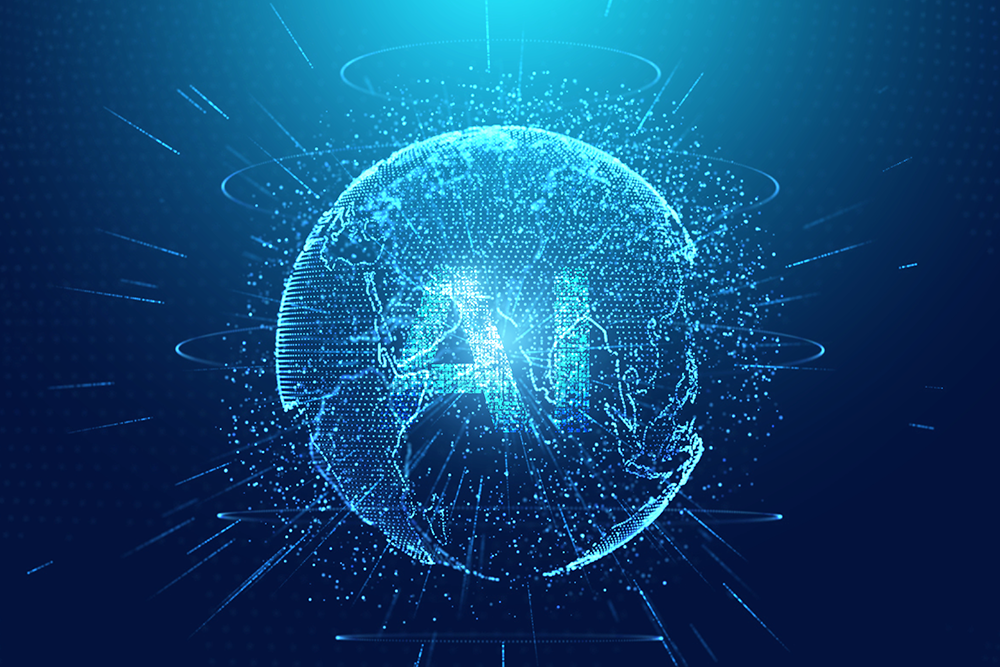
Banks, Credit Institutions, Utilities all face the same challenge. When a customer is going past due in his obligations, they engage in a series of activities with the aim to collect the past due amount.
This process is organized through a strategy that comprises of a predefined schedule of SMS, emails, letters, phone calls, field visits, assignments to external agencies, and legal actions. This schedule differs from creditor to creditor, as it is based on each creditor’s business strategy and must be aligned with local legislation and rules of conduct.
While the available software solutions permit us to optimize the usage of all the above tools in order to sequence the contacts with the customers through the different channels, they do not address several fundamental issues:
Certainly, as our knowledge develops, the strategies become more “informed” and results can be ameliorated. However, this process is long and costly, both in terms of analysis spend time and of lost opportunities.
For example, making a call to the customer that is past due, is always considered a crucial step in the collections process as we expect that the human interactions will generate a promise to pay from the customer. Not calling on time, a debtor who needs human persuasion, increases, even more, the risk of non-repayment as the delinquency amount becomes bigger and bigger. In the same terms, calling a debtor who is not cooperative is a wasted effort. Also, when shall we call, is there a time in the day that is preferable to others?
On questions like this, the usage of Big Data and Machine Learning can permit us to immediately increase the expected results.
LAZY PAYERS / SELF-CURED
We all know that between our customers there is a certain group that is not really an issue for collections. They can be defined as a “group of customers, that despite having the financial capacity, they do not usually give strong attention to the billing date of their obligation. Thus, they may exceed the payment date (not having a specific periodicity on this action), but at the end will finish by paying their past due”.
We usually characterize those as lazy payers and also as self-cured customers.
Our first challenge is how to identify those. By using extensive data sets of previous customer’s history and performance, and the creation of a new set of metrics, A.I. models are permitted to predict the “lazy payers” customers in a given portfolio, with an accuracy of 98%-99%. Additionally, this prediction shows good stability in time with day-to-day stability superior to 99,5%.
What are the implications of this?
Creditors can decide to exclude those customers from the calling lists, in their existing collections strategy, at least for the period that the model considers that the payment of the past due from the lazy payer will be made.
Such a decision will reduce the total number of calls to the customers by up to 50% thus maintaining the same level of collected amounts.
This permits a significant redistribution of the effort towards customers that necessitate human interaction to pay for their past dues.
Defaulters
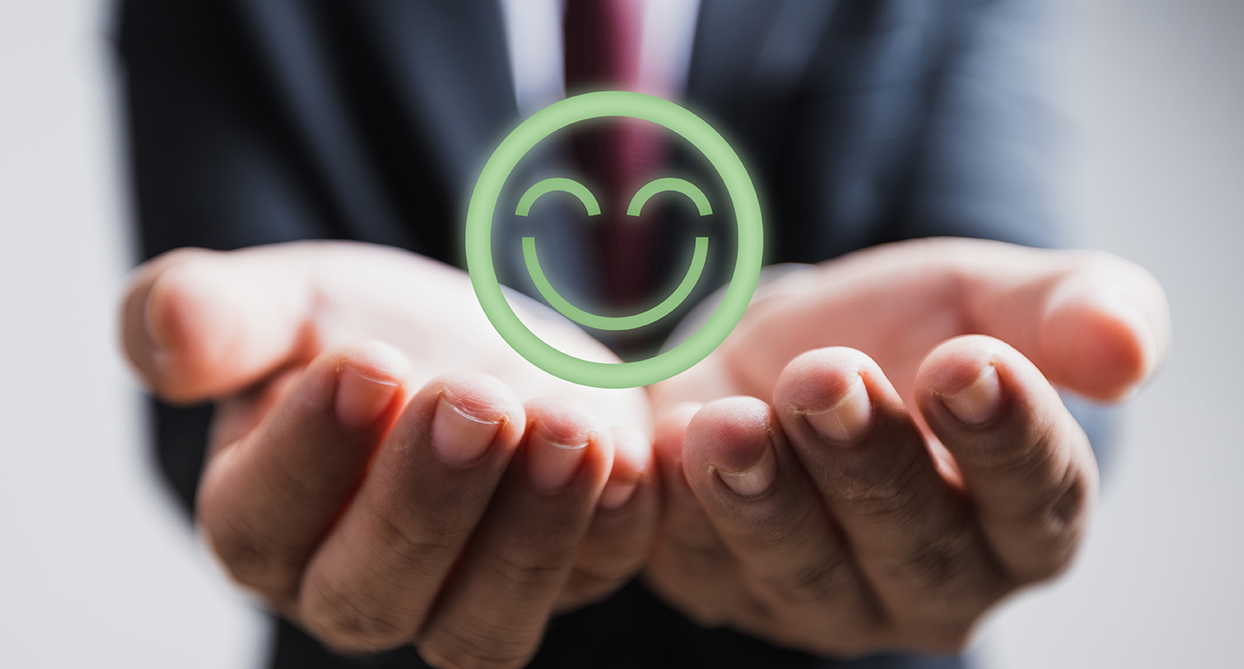
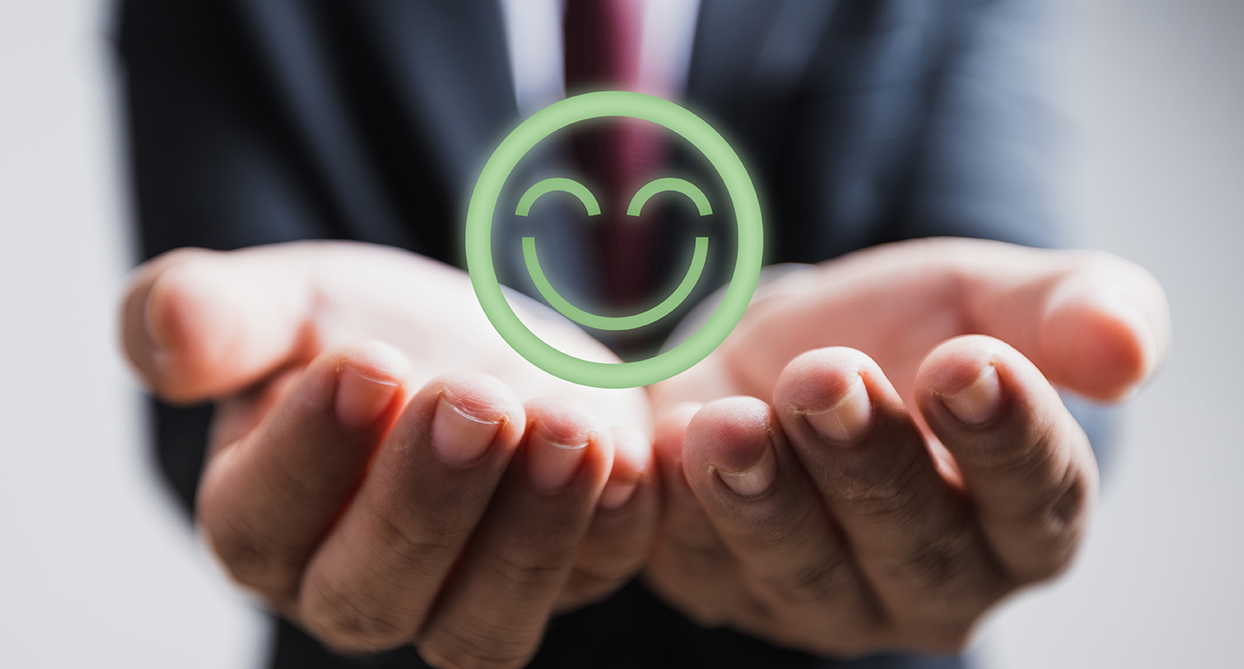
All Collections Managers would like to know well in advance which of the customers that are entering collections will default at a certain time.
By the usage of the same model it has been possible to identify with a very high prediction rate, close to 96% those between the customers that are currently in early collections that will be defaulting at a future stage.
This indicator supports the optimization of the strategy in place in order to prioritize actions on the “defaulters” segment before those have arrived at the default stage, as this has been legally set according to the legislation in place and to the policy of the organization.
The expected business result is a reduction in the amount that will default, by preventive actions, thus minimizing the loss that the Creditor will suffer at the end.
FUTURE EXPLORATIONS
In the near future, we expect that through the usage of A.I. and ML techniques further developments and new models will be applied that will permit to increase the effectiveness of the collections strategies.
The first indicators at the customer level enable the design of an “intelligent” segmentation of the database. This allows the adjustment of strategies and tools in accordance with the customer’s behavior in a more dynamic and informed way. Grouping customers into homogeneous segments aims at identifying patterns/trends, discovering knowledge, and building behavioral scoring models per segment from a risk management point of view. Low-risk, medium-risk, and high-risk customers need personalized treatment plans for high-quality management. Intelligent segmentation can identify the most significant customer characteristics for dividing customers into segments and highlighting the interval into which values of customer characteristics belong per segment to differentiate segments and create rules.
Specific prediction problems as the right time to call, responsiveness to alternative collections channels (i.e., recommend treatment plans in a next-best-action context), the probability of getting a promise, the kept promise ratio, assignment optimization with respect to agent performance/profile, and subject to constraints (e.g., available working hours, working days, working channels, maximum number of cases per agent, agent seniority level, etc.), early warning signals (i.e., detect abnormal behavior in the debt collection processes) are between those that we expect the major breakthroughs to take place.
Our business will become more “informed”, results are going to be more predictable, diving debt collections intelligence to new highs.
Interested in exploring an A.I. problem?
Get in touch to arrange a meeting with our A.I. team.